Improving generative AI models for real-world medical imaging
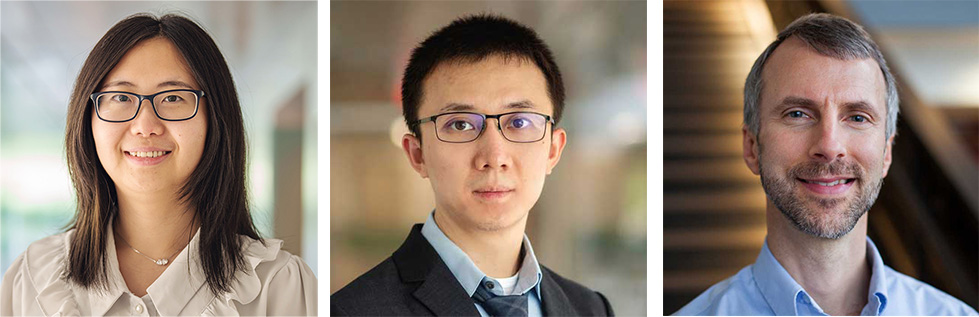
Professors Liyue Shen, Qing Qu, and Jeff Fessler are working to improve a type of deep generative models known as diffusion models. These models are highly successful in applications such as image generation and audio synthesis, as well as medical imaging and molecule design.
“It is quite exciting to explore the potential of generative models in medical imaging and other scientific disciplines,” Shen said. “I am particularly excited to work on developing new and more efficient diffusion models that can surpass the current limitations.”
It is quite exciting to explore the potential of generative models in medical imaging and other scientific disciplines.
Prof. Liyue Shen
Diffusion models are designed to learn the data distribution, which is important for understanding large-scale and complex real-world data. The team is specifically examining how diffusion models could be applied to inverse problems, which is when a set of observations are used to determine what factors produced the end results.
“Generative models are one of the hottest topics in machine learning right now, and I’m excited to have the opportunity to investigate their potential for solving inverse problems, especially in medical imaging,” said Fessler, the William L. Root Collegiate Professor of EECS. “We’re hoping to apply the methods developed in this project to large-scale 3D medical imaging applications, like low-dose X-ray CT and accelerated MRI.”
We’re hoping to apply the methods developed in this project to large-scale 3D medical imaging applications, like low-dose X-ray CT and accelerated MRI.
Prof. Jeff Fessler
Currently, there are many limitations regarding the practical applications of diffusion models. In particular, the training and inference of diffusion models are both data-intensive and computationally demanding, which limits their use in many scientific disciplines.
“In real-world medical imaging, the images are always high-resolution and high-dimensional, which is far beyond what can be handled by the existing diffusion models regarding memory and time efficiency,” Shen said. “In addition, the inference time of diffusion models is undesirably long because of the iterative sampling procedure.”
The team is working to improve the practical applicability and mathematical interpretability of diffusion models by developing new architecture design and latent embeddings. They’re also developing new techniques to improve the training and sampling efficiency of diffusion models, and they’re working to create computationally efficient diffusion models for high-dimensional data that could further enhance data, memory, and time efficiency. This could greatly improve applications such as high-dimensional, high-resolution biomedical imaging, as well as motion prediction that’s based on high-dimensional dynamic imaging.
“Diffusion-based generative models are poised to majorly influence scientific fields. However, implementing these models for scientific discovery presents challenges such as ensuring interpretability, robustness, trustworthiness, and fairness,” Qu said. “We aim to develop a deeper mathematical understanding of these models to guarantee controllable and trustworthy data generation process.”
We aim to develop a deeper mathematical understanding of these models to guarantee controllable and trustworthy data generation process.
Prof. Qing Qu
The research received funding from the Michigan Institute for Computational Discovery and Engineering, a unit within the Office of the Vice President for Research.