Machine learning proves useful for analyzing NBA ball screen defense
The team used machine learning to extract information from NBA sports data for automatically recognizing common defense strategies to ball screens.
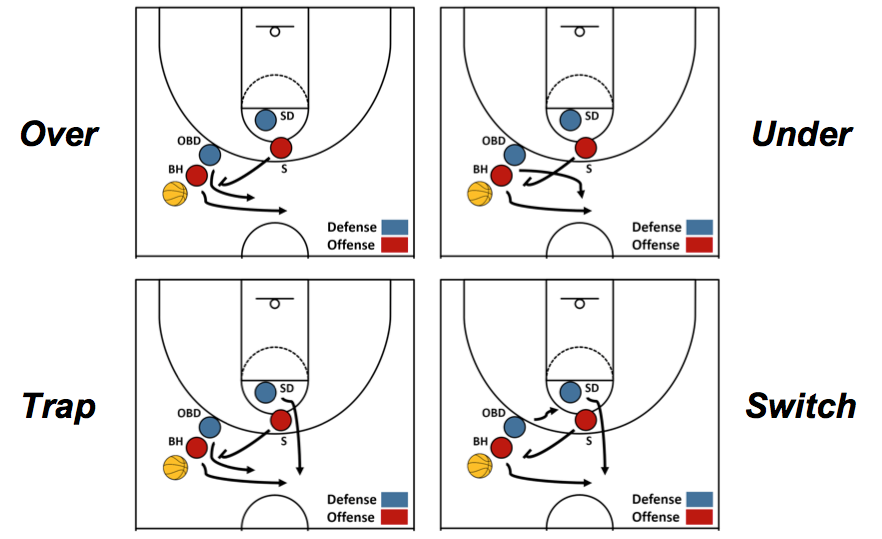
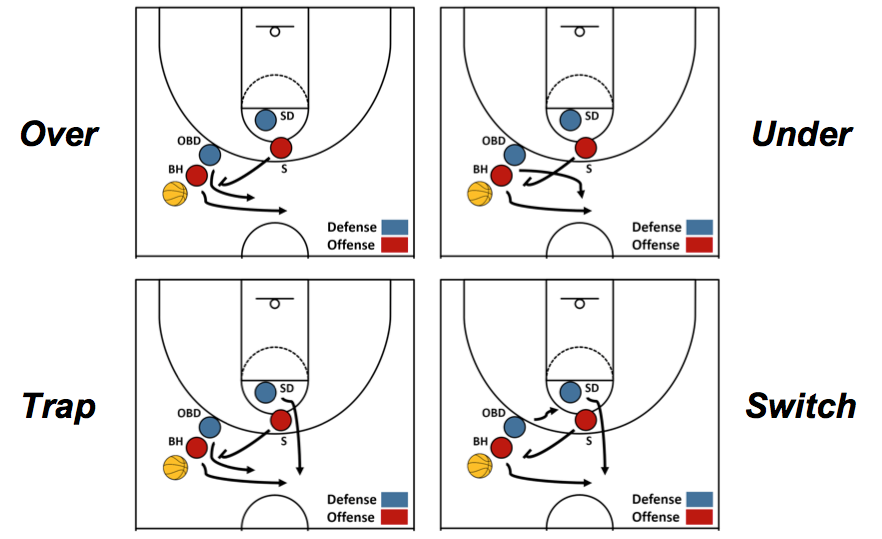
A research team including Avery McIntyre (December 2015 alumnus of the undergraduate CS program at Michigan) and Prof. Jenna Wiens has used machine learning to extract information from NBA sports data for automatically recognizing common defense strategies to ball screens. They shared their findings at the 10th MIT Sloan Sports Analytics Conference in Boston, MA, which took place on March 11–12, 2016.
The researcher’s paper, “Recognizing and Analyzing Ball Screen Defense in the NBA” was selected as the top paper in the “Basketball track” of the research paper competition. Using data from multiple seasons and supervised machine learning techniques, the researchers were able to categorize the defense of the NBA’s most popular offensive action in four ways: over, under, switch, and trap. They presented observations and trends at both the team and player levels and state that “Our work is a step towards the construction of a coaching assistance tool for analyzing one of the game’s most important actions.”
News of the paper’s findings was reported on by media including in articles by ESPN, Sports Illustrated, and FiveThirtyEight.
Prof. Wiens’ primary research interests lie at the intersection of machine learning and healthcare. The overarching goal of her research agenda is to develop the computational methods needed to help organize, process, and transform data into actionable knowledge. In addition to her work in healthcare, she develops machine learning methods for the extraction of strategically useful information from player tracking data in the National Basketball Association.
Prof. Wiens received her PhD in Electrical Engineering and Computer Science from the Massachusetts Institute of Technology in 2014 and joined the faculty of CSE at the University of Michigan that year. Her PhD research focused on developing accurate patient risk-stratification approaches that leverage spatiotemporal patient data, with the ultimate goal of discovering information that can be used to reduce the incidence of healthcare-associated infections. Prof. Wiens was awarded an NSF CAREER Award in 2016. She is affiliated with the Artificial Intelligence Laboratory at Michigan.