Two projects recognized with JP Morgan Chase Faculty Research Award
Two projects from U-M researchers have been recognized with the JP Morgan Faculty Research Award, which funds study into advancements in artificial intelligence with particularly applicable uses in industry.
Following is a summary of each of the recognized projects.
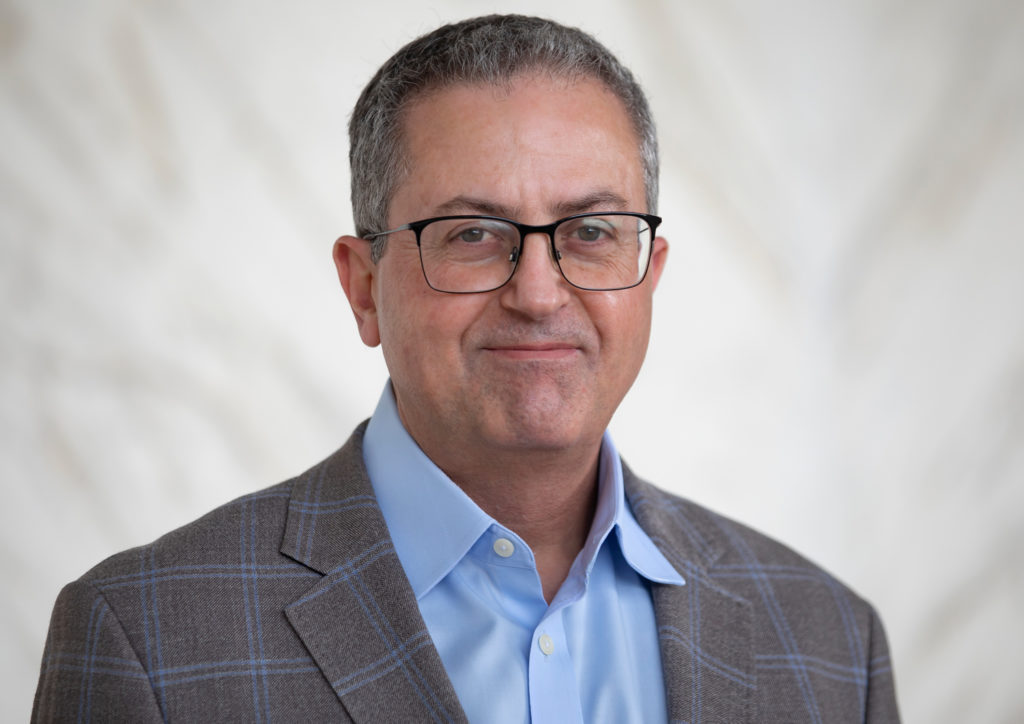
“Strategic Modeling of Fraud on the Payments Network”; Led by Lynn A. Conway Professor of Computer Science and Engineering Michael Wellman
The researchers propose to build on a prior project, development of an agent-based simulation platform to study salient strategic questions about financial payment networks. This platform has already been applied to two different domains: strategic debt compression and adoption of real-time payments. In this project, their focus is on credit fraud, specifically understanding implications on the pattern and deterrence of fraud based on availability of advanced detection technologies.
Michael Wellman is the Richard H. Orenstein Division Chair of Computer Science and Engineering
Lynn A. Conway Collegiate Professor of Computer Science and Engineering. His research focuses on uses of artificial intelligence in electronic commerce.
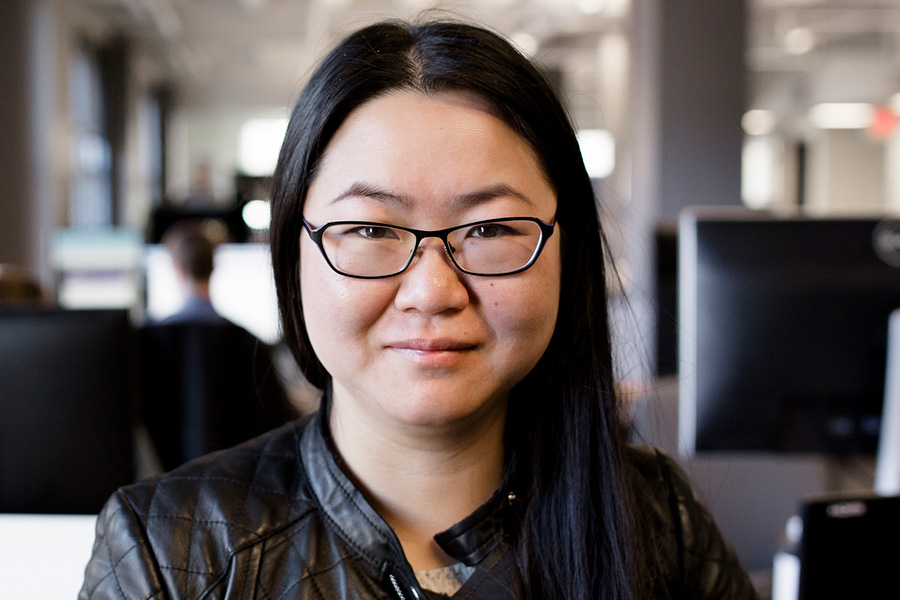
“Towards Personalized Intelligence at Scale”; Led by Prof. Lingjia Tang and Prof. Jason Mars
Personalized Intelligence (PI) aims to provide truly customized AI experiences tailored to each individual user. It is well known that personalization provides a superior user experience. In many applications, personalized AI models may even be required due to the high degree of heterogeneity in the user population. However, in industry, we typically adopt the “one model serves all” approach, training a single massive model to serve all users. An example of when new customized models are needed is when there are emerging new classes added to the classifier due to individual user needs. The state-of-the-art approach is then fine-tuning the pre-trained model to create a personalized model. However, having a separate personalized model for each user in this approach would incur significant training and memory consumption costs in production that prove prohibitive for large models.
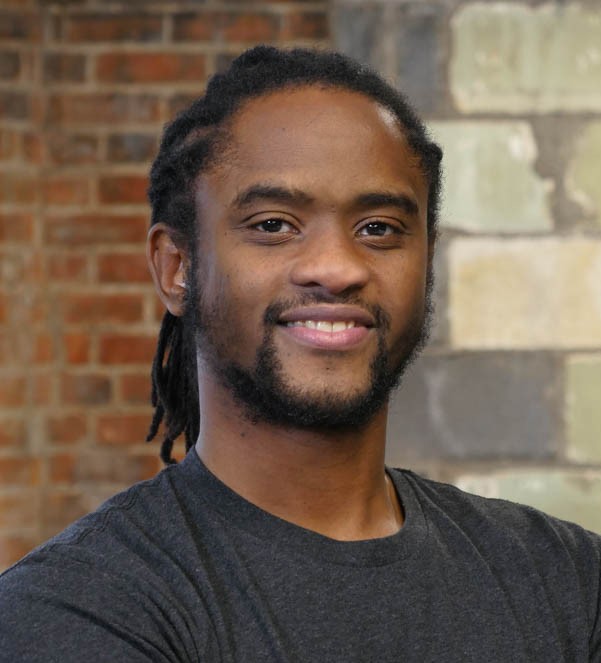
In this work, the researchers propose a novel model architecture and training/inference framework to enable Personalized Intelligence at scale, aiming to provide customized models to millions of users with much lower training and memory cost. They plan to achieve this by designing and attaching a Personalization Head (PH) to pre-trained models, such as a large general language model (LM). The PH is designed to capture the knowledge and needs specific to the user. During training, the base LMs are frozen and only the parameters in PH are updated and are unique per user. This results in significantly smaller training cost than traditional fine-tuning approaches when scaled across many users. Their preliminary results already demonstrate a significant accuracy improvement with customized models, with lower training costs than fine-tuning general models.
Prof. Lingjia Tang researches Computer architecture and compiler and runtime systems, specifically large scale data centers. Prof. Jason Mars focuses primarily on cross-layer systems architectures for emerging applications, datacenter and warehouse-scale computer architecture, and hardware/software co-design.